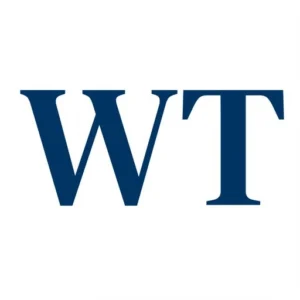
During a visit to Microsoft’s headquarters in Seattle in May, Chanel CEO Leena Nair and her leadership team were exploring how AI could revolutionize the luxury sector. Their discussions with tech giants, including Microsoft, focused on tapping into AI’s potential to enhance creativity and business practices. However, what happened next during their interaction with ChatGPT—a tool developed by OpenAI—took an unexpected turn.
When Nair asked the AI to generate an image of Chanel’s senior team at Microsoft, the result was not only inaccurate but also somewhat comical. The AI presented an image of an “all-male team in suits,” a stark contrast to Chanel’s actual diverse leadership team. Nair, who has been a trailblazer for women in business, particularly as the first female Chief HR Officer at Unilever, was quick to point out the error. In her own words: “This is Chanel. Yes, 76% of my organization is women, 96% of my clients are women, female CEOs.” With a touch of humor, she added, “This is what you’ve got to offer, ChatGPT? Come on.”
This lighthearted moment quickly became a talking point across the media, highlighting the troubling issue of AI bias – something that, despite being built on algorithms and data, is far from immune to human prejudices and stereotypes.
What is AI Bias?
AI bias is the tendency of artificial intelligence systems to make unfair or imbalanced decisions, often because the data used to train them reflects existing social biases. AI learns from vast amounts of data, and if that data is flawed or skewed by historical inequalities, the AI may unintentionally reinforce these issues. This can lead to outcomes that disproportionately affect certain groups, with serious real-world consequences.
Examples of AI Bias in Action
Several high-profile examples of AI bias have already made headlines in recent years, serving as stark reminders of how these technologies can perpetuate harmful stereotypes.
In 2015, researchers from Carnegie Mellon University examined Google’s advertising algorithms and found that certain job ads for high-income positions were shown to male users more often than to female users, reinforcing gender disparities in the job market.
Another case occurred in 2014 when Amazon introduced an AI tool designed to eliminate bias in its recruitment process. Unfortunately, the tool ended up penalizing resumes that included words such as “woman” or referred to female-oriented roles and showed a clear preference for resumes with traditionally masculine language. By 2018, Amazon had scrapped the tool, acknowledging that it was perpetuating gender bias.
More recently, iTutorGroup, a global education company, found itself entangled in a discrimination lawsuit. Its AI-driven application review system was found to discriminate against older female candidates aged over 54 and men over 60. The company eventually settled for $365,000 after the Equal Employment Opportunity Commission (EEOC) investigated the claims.
How Can AI Bias Be Overcome?
Addressing AI bias requires intentional action and transparency from organizations developing and using these systems. Here are some steps that can help reduce bias in AI technologies:
Establish Clear Guidelines:
Organizations must set clear rules and guidelines for identifying and mitigating bias in their AI systems. This should include processes for recognizing when bias might be creeping into data, models, or decision-making, and how to address it. Companies must document these occurrences and make the efforts to counteract bias transparent to the public.
Ensure Representative Data:
One of the main sources of AI bias is flawed or unrepresentative data. Before training an AI model, organizations must carefully examine what constitutes a truly representative dataset. This means understanding the population the model is intended to serve and ensuring the data reflects diverse experiences and perspectives.
Document Data Selection and Cleansing:
Bias often arises during the data selection and cleaning processes. To avoid this, organizations should be transparent about how they select and process their data. By documenting these steps and making them open to scrutiny, businesses can help ensure that any potential sources of bias are flagged and addressed early.
Screen Models for Bias:
When evaluating AI models, it’s not enough to only focus on accuracy and precision. Organizations should also include checks for bias as part of their model assessment process. A model that performs well on the metrics of accuracy may still fail on fairness, and identifying these gaps early can prevent problems down the line.
Ongoing Monitoring and Review:
Finally, AI systems must be continuously monitored once they are in operation. What works well in theory during testing can perform very differently in the real world, so organizations need to track the ongoing performance of AI models to spot any emerging biases. Regular audits and reviews will ensure that any unanticipated issues can be addressed before they cause serious harm.
AI’s potential is undeniable, but as these examples show, it’s important to address its inherent biases. If we fail to tackle these issues head-on, we risk reinforcing existing inequalities rather than overcoming them. The conversation around AI bias, sparked by incidents like the one with Leena Nair, is vital for driving change. With increased awareness and proactive steps, we can build AI systems that are fairer, more inclusive, and better equipped to serve a diverse world.